Insisting on AI that is Transparent, Fair, and Accountable
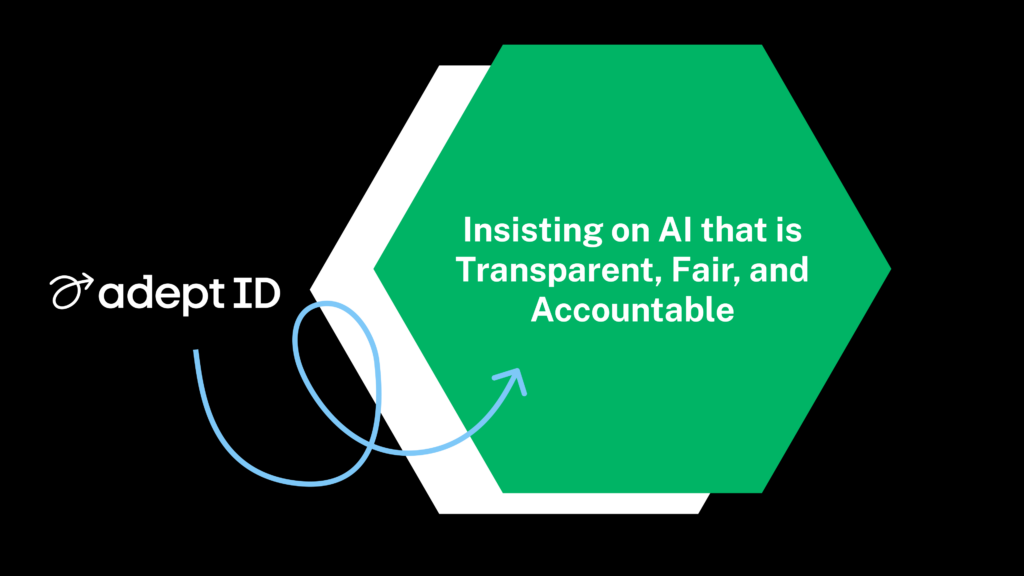
Over the next several weeks, we’ll be sharing our perspective on how AI can be built responsibly to serve the Talent ecosystem. Here are our opening thoughts.
An Invitation: Let’s Solve Our Shared Problem Together
To increase opportunity, inclusion, and productivity in the talent sector, we must insist on AI models that meet and exceed high expectations for transparency, fairness, and accountability.
Problem Overview
The market for Talent has a tragic mismatch. Much of the workforce is underrecognized and underemployed. Meanwhile, employers experience critical shortages. Typical hiring processes overvalue credentials, fail to identify potential, and harm individuals and groups through bias. AI models seem an obvious part of the solution, and interest has intensified for uses including career navigation for jobseekers and talent identification for employers and trainers. For many, AI’s potential has been marred by its checkered history of bias and inaccuracy. As regulatory scrutiny evolves, we lack a consensus about what we expect AI to do, and how to ensure that it does well.
Invitation
The solution is to build and use more and better AI, while advocating that all others do so. Arms-length government regulation is not enough: we need authentic, shared oversight. We invite makers and users to advance shared accountability and to build and use AI to make hiring decisions that recommend better candidates and reduce bias, by observing these four transparency practices.
AdeptID uses fair and transparent approaches to artificial intelligence (AI) to get more people into better jobs, faster. With partners like OneTen, Year Up, Jobs for the Future, and Multiverse, we use our technology to reduce the barriers faced by workers, jobseekers, students, educators, and employers. We address this open letter to our peers: AI builders and users, and to all those who share our interest in ensuring that AI fosters a more inclusive and dynamic labor market.
Building and Using AI the Right Way: Four Practices of Transparency
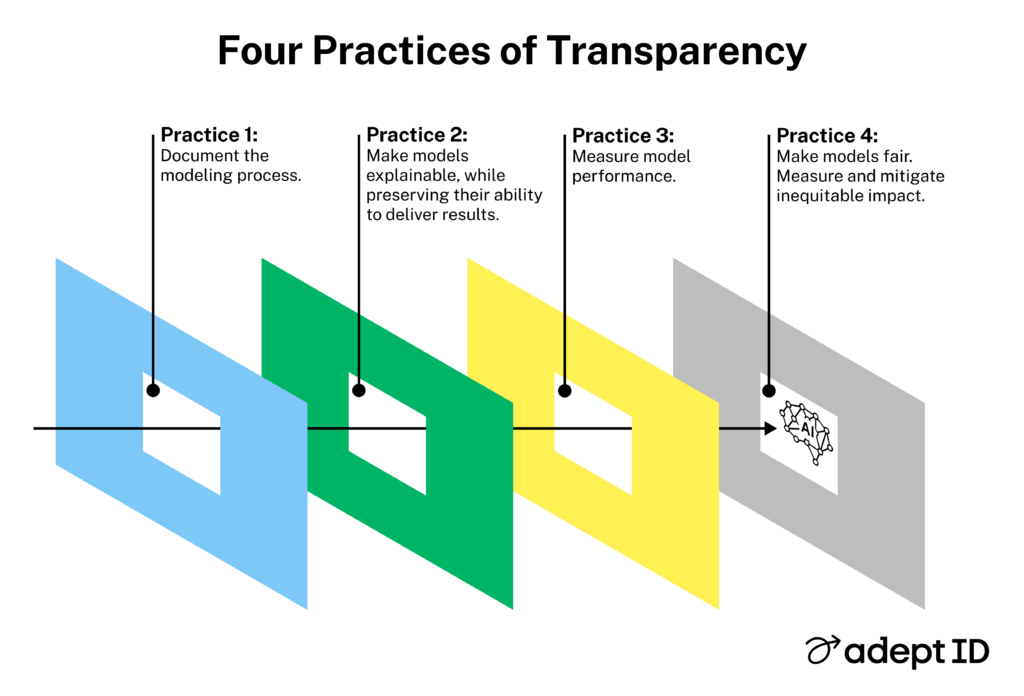
Practice 1: Document the modeling process.
Users need to know how a system was built and how it works. What algorithms are used? On what data is the model trained? How is bias being mitigated? Makers who articulate their modeling choices give users invaluable maps. Enabling users to interrogate results builds user knowledge and confidence. Offering open documentation, a bedrock of our practice at AdeptID, invites technical and non-technical users to evaluate what they are buying or using, and to build their AI expertise.
Practice 2: Make models explainable, while preserving their ability to deliver high-quality results.
People impacted by a model should be able to see why it arrives at a recommendation, and AI makers should share accessible explanations. These explanations can be achieved from an outcomes-oriented perspective without sacrificing model quality. At AdeptID, we share frequent ‘under the hood’ analyses of how our model works. Users learn germane things, like which job candidate characteristics lead to a given recommendation. This practice gives diverse stakeholders clarity on how a model works without sacrificing impact.
Practice 3: Measure model performance.
No one would license a stock-picking algorithm without first seeing quantitative evidence that it was good at picking stocks. However, such a norm does not yet exist for AI in hiring. Developers routinely offer models without evidence of results. Buyers and users should treat such a failure to share performance as unacceptable. All AI makers should produce routine evidence of model results, such as impact on hiring goals. At AdeptID, we share such test results publicly, including showing just how much improvement we still have to do!
Practice 4: Make models fair. Measure and mitigate inequitable impact.
- Test models for fairness. Does a model hire qualified men over qualified women? Does it make fewer errors with white and Asian candidates, rejecting them for unsupported reasons less often than it does for Black and Hispanic candidates?
- Use more demographic data, not less. Many stakeholders are wary of sharing demographic data lest it lead to biased treatment. But carefully curated demographic data enables us to track differences in outcomes and actively mitigate bias. In work with employer coalitions, AdeptID has accomplished this with a derivative of Microsoft’s excellent Fairlearn toolkit.
- Involve and empower all stakeholders. AI buyers and users: ask makers tough questions about data, outcomes, and fairness. Developers: make tests continuous in models. Users and makers: provide guidelines to help students and jobseekers get fair treatment.
Co-Design Authentic Shared Oversight
So far, there is very little regulation of AI, especially in the talent sector. That will soon change, and rightly so. At this moment, users and makers have a chance to work with public agencies and auditors to build a shared accountability system. By building a common set of expectations, we can protect jobseekers, tap industry expertise, avoid rules that slow down hiring and job mobility, and encourage government, producer, and consumer ownership of the regulatory process. Here are five steps public/private partners should take whether or not they are specifically required by regulators:
- Make accountability systems that monitor models continuously, not annually.
- Promote fairness and transparency without slowing talent’s efforts to get jobs.
- Organize Independent third-party auditors who meet rigorous standards.
- Impose sanctions for the harmful use of AI.
- Encourage users and buyers to refuse models that fail to perform fairly and transparently.
Continuation…
Let’s get more people into better jobs, faster by creating and insisting on AI practices that are transparent, fair, and accountable. If you’re hungry, like we are, for dialogue oriented around action, let’s connect.