Transparent Practice #3: Measure model performance
Over the past few weeks, we shared an Open Letter insisting on AI that is Transparent, Fair, and Accountable for job seekers, employers, and training providers, and we’ve detailed two of our four recommended processes of transparency.
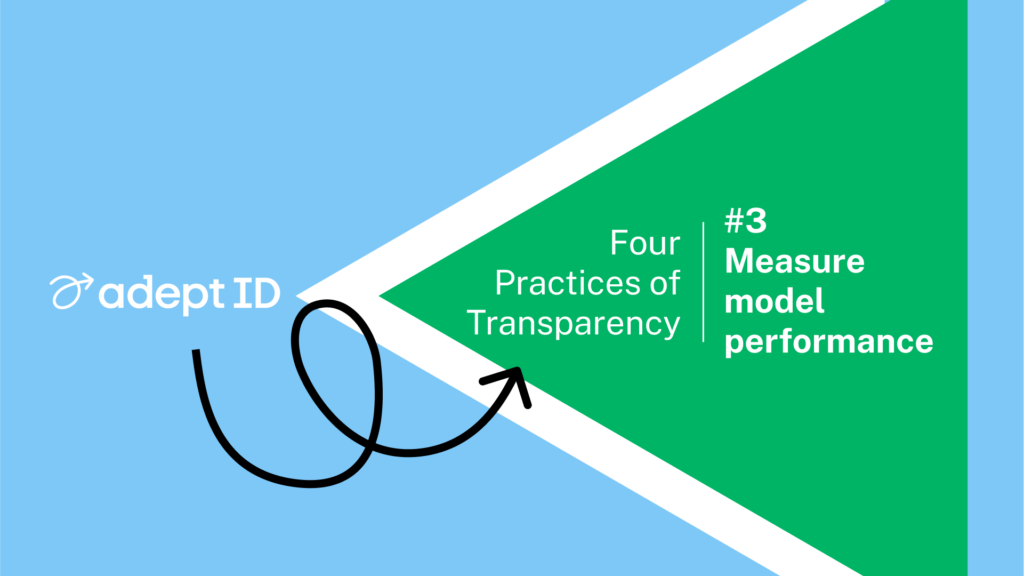
The two practices we’ve already shared – documenting how a model is made and can be used, and making sure its results are explainable – lead to our third required practice for transparency: measuring the actual performance of the model.
It is amazing how little attention is currently paid to the performance of models in the talent sector. No one would license a stock-picking algorithm without first seeing quantitative evidence that it was good at picking stocks.
In the Talent/HR space, there is no norm or expectation for similar evidence. Developers routinely market models for which they lack demonstration of quality or results protection of fairness. Buyers and users should treat this vacuum of performance accountability as unacceptable.
At a minimum, all AI developers should produce routine evidence of the results of their model, so that model performance can be understood. AI-based products should provide evidence showing the impact of the model on hiring goals, such as improved matching of candidates with skills required, or increased reliance on the evidence of promise or potential, rather than on credentials, traditional career paths, or degrees.
At AdeptID, we run these evaluations on our models, and we conduct tests that are integral to our model design and improvement work. In addition to applying what we learn to upgrade models and overcome problems, we also share testing results with the public, even when it’s clear that there’s room for improvement. Sometimes that involves educating our customers on accuracy metrics (like Receiver Operating Characteristics), but this education is part of ensuring quality and integrity.
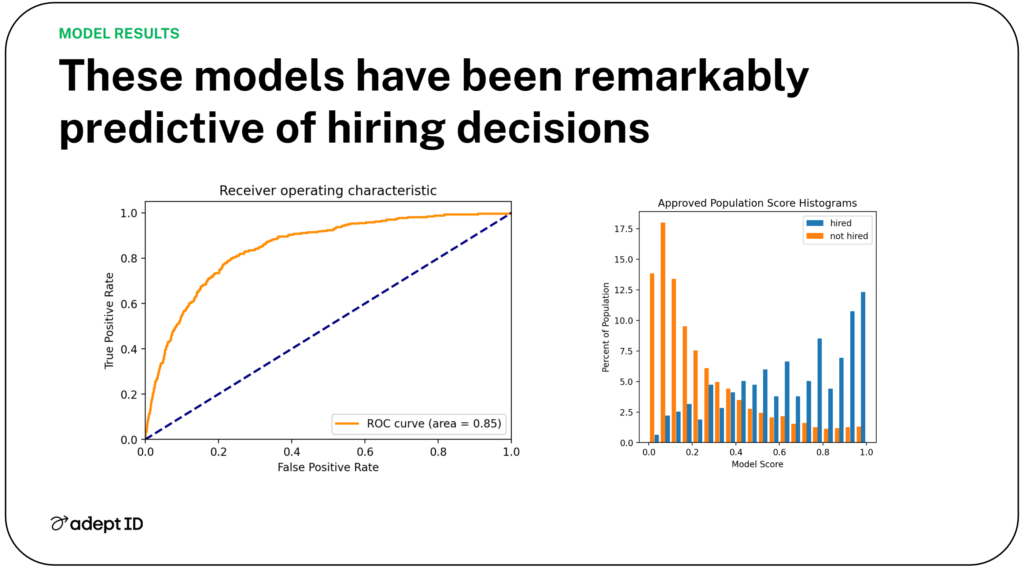
The diligent critiquing and sharing of a model’s results give users practical ways of assessing the model and holding its developers accountable. It also powers the ongoing learning of the developer and their partners.